Translate this page into:
In silico study of potential SARS-CoV-2 antagonist from Clitoria ternatea
Address for correspondence: Yin-Quan Tang, Impact Lab (Medical Advancement for Better Quality of Life), Taylor’s University, Subang Jaya, Malaysia. E-mail: yinquan.tang@taylors.edu.my
This is an open-access article distributed under the terms of the Creative Commons Attribution-Noncommercial-Share Alike 3.0 Unported, which permits unrestricted use, distribution, and reproduction in any medium, provided the original work is properly cited.
This article was originally published by Qassim Uninversity and was migrated to Scientific Scholar after the change of Publisher.
Abstract
Objectives:
In this study, we implemented a structure-based virtual screening protocol in search of natural bioactive compounds in Clitoria ternatea that could inhibit the viral Mpro.
Methods:
A library of twelve main bioactive compounds in C. ternatea was created from PubChem database by minimizing ligand structure in PyRx software to increase the ligand flexibility. Molecular docking studies were performed by targeting Mpro (PDB ID: 6lu7) via Discovery Studio Visualiser and PyRx platforms. Top hits compounds were then selected to study their Adsorption, distribution, metabolism, excretion, and toxicity (ADMET) and drug likeness properties through pkCSM pharmacokinetics tool to understand the stability, interaction, conformational changes, and pharmaceutical relevant parameters.
Results:
This investigation found that, in the molecular docking simulation, four bioactive compounds (procyanidin A2 [−9.3 kcal/mol], quercetin-3-rutinoside [−8.9 kcal/mol], delphinidin-3-O-glucoside [−8.3 kcal/mol], and ellagic acid [−7.4 kcal/mol]) showed producing the strongest binding affinity to the Mpro of severe acute respiratory syndrome coronavirus 2, as compared to positive control (N3 inhibitor) (−7.5 kcal/mol). These binding energies were found to be favorable for an efficient docking and resultant. In addition, the stability of quercetin-3-rutinoside and ellagic acid is higher without any unfavorable bond. The ADMET and drug likeness of these two compounds were found that they are considered an effective and safe coronavirus disease 2019 (COVID-19) inhibitors through Lipinski’s Rule, absorption, distribution, metabolism, and toxicity properties.
Conclusion:
From these results, it was concluded that C. ternatea possess potential therapeutic properties against COVID-19.
Keywords
Computational screening
coronavirus disease 2019
molecular docking
phytochemical
severe acute respiratory syndrome coronavirus 2
Introduction
The outbreak of coronavirus disease 2019 (COVID-19) has widely affected the whole world. This infectious disease that targets the respiratory system is caused by a novel Betacoronavirus, the 2019 novel coronavirus or known as severe acute respiratory syndrome coronavirus 2 (SARS-CoV-2).[1,2] Fever, cough, fatigue, rhinorrhea, and dyspnea are the symptoms of COVID-19 infection.[2] However, it has been stated that the number of asymptomatic COVID-19 patients was higher than number of symptomatic patients, with the prevalence of asymptomatic patients slightly higher for people older than 50.[3] The World Health Organization has reported 290,959,019 cases and 5,446,753 deaths caused by this disease globally as of January 2022.[2] Therefore, more studies should be carried out to search for effective therapeutic or preventive measures to stop the spread of this massive outbreak.
Coronaviruses (CoVs) are single-stranded positive-sense RNA large-sized viruses (27-32 kb) and SARS-CoV-2 is about 29.9 kb in size.[4] The virus contains four structural proteins including spike protein (S), envelope protein (E), membrane protein (M), nucleocapsid protein (N), and 16 non-structural proteins (nsp1-16).[5] The enzyme that plays an important role in processing polyproteins (pp) which synthesize these structural and non-structural proteins is main protease (Mpro), a chymotrypsin-like hydrolase (3CL hydrolase). Mpro is responsible for the maturation of main functional enzymes such as replicase and helicase, mediating the viral gene replication and transcription. Thus, it is a suitable active target for the anti-CoV drugs.[5-7]
Computational screening is one of the important and suitable ways to develop new antiviral drugs from existing natural compounds to reduce the cases and death of COVID-19 pandemic.[8-10] Molecular docking is a virtual computational method commonly used to predict the complex of two binding molecules including biological macromolecules such as protein, DNA and RNA, or small molecules such as endogenous ligands and drugs.[6,11] Small-molecule docking can be used to determine ligand interactions with target protein in structure-based drug development. The orientation of the small ligand molecule inside the target protein’s binding cavity is shown and a specific scoring function will evaluate the resulting docking pose. The scores are generated for each pose and the ranking can be determined by the values of different poses and ligands.[12] Binding affinity is one of the essential parameters regarding protein-ligand interaction that can assess the strength of interaction between a drug-target pair.[13]
Plants or natural products contain a lot of health beneficial bioactive compounds. Clitoria ternatea, commonly known as butterfly pea flower or telang tree in Malaysia is a long-lived perennial herb from the Fabaceae family, which is distributed in tropical or subtropical regions.[14-16] It is usually 90–162 cm tall with vivid deep-blue and white colored flowers as well as six to eight brown or black colored seeds in every pod. Butterfly pea flower is widely used as a natural blue coloring in drinks and foods in Malaysia such as Nasi Kerabu, Pulut Inti, and Pulut Tatai. Livestock also prefer it as a source of food compared to legumes due to its mild and acceptable taste.[14,15] The flowers contain several phytochemicals including phenolic compounds, anthocyanins, and flavanols. Studies proved that this flower possesses different medicinal effects including antioxidant, antihyperglycemic, antihyperlipidemic, antimicrobial, and hepatoprotective.[15-18] For example, a study conducted proved that green synthesized selenium nanoparticles using C. ternatea showed significant antibacterial activity against Staphylococcus aureus and C. albicans.[19] Hence, butterfly pea flower was chosen in this study for COVID-19 drug research. The aim of this study was to examine potential anti-CoV activities of active compounds in C. ternatea using structure-based virtual screening approach.
Methods
Ligand and protein preparation
A library of twelve C. ternatea’s main bioactive compounds were obtained from PubChem in.sdf format.[15] The three-dimensional (3D) crystal structure of SARS-CoV-2 Mpro (PDB ID: 6lu7) was downloaded from Protein Data Bank (www.rcsb.org) and the resolution was 2.16 Å.
Molecular docking simulation
Discovery Studio Visualiser was used to determine the active sites of the receptor Mpro, which are His41, Met49, Phe140, Gly143, His164, Met165, Glu166, Leu167, Pro168, His172, Gln189, Thr190, and Ala191 as shown in Figure 1 and Table 1. The water molecules as well as N3 inhibitor ligands from Mpro were removed and polar hydrogens were added to the structure. Software PyRx was used to perform molecular docking using Mpro as receptor and the compounds of C. ternatea that were chosen as ligands. AutoDock Vina was used to minimize ligands energies. The identified active sites from 2D diagram of Mpro were chosen into the grid box before running the docking. N3 is known for its specificity inhibit Mpro from multiple CoVs, including SARS-CoV and MERS-CoV,[20-23] was used as positive control in this study. N3 inhibitor’ structure was obtained from ChemSpider. Finally, the 2D interactions and bonds at the binding active site were shown in Discovery Studio Visualiser.
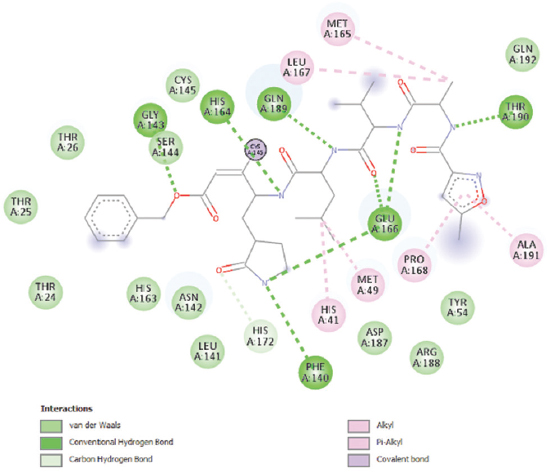
- 2D diagram of interaction of active site of Mpro with N3 inhibitor

Adsorption, distribution, metabolism, excretion, and toxicity (ADMET) and drug likeness prediction
ADMET was predicted for the four compounds with strong binding affinities which are procyanidin A2, quercetin-3-rutinoside, delphinidin-3-O-glucoside, and ellagic acid. The SMILES of the compounds were obtained from PubChem and uploaded onto pkCSM pharmacokinetics tool (http://biosig.unimelb.edu.au/pkcsm/prediction).
Results
Binding affinity score of bioactive compounds in C. ternatea targeting Mpro
In the library of twelve main bioactive compounds in C. ternatea, there are three main classes: Flavonoid, anthocyanin and phenolic acid. The docking scores are shown in Table 2 according to their binding affinities scores. Based on Table 2, both flavonoid and anthocyanin classes showed stronger binding affinity targeting Mpro than phenolic acid.
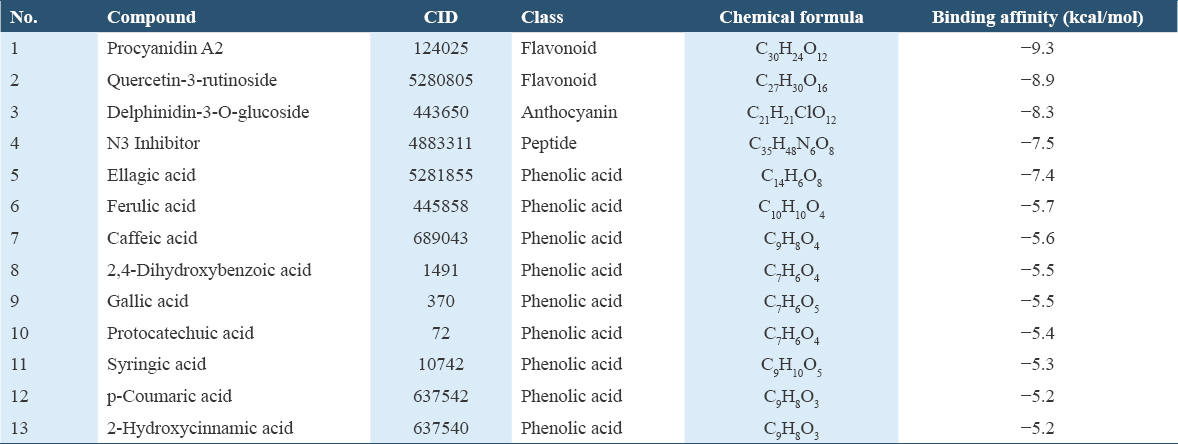
Identification of SARS-CoV-2 antagonist phytocompounds
In the molecular docking simulation, four bioactive compounds (procyanidin A2 [−9.3 kcal/moL], quercetin-3-rutinoside [−8.9 kcal/moL], delphinidin-3-O-glucoside [−8.3 kcal/moL], and ellagic acid [−7.4 kcal/moL]) showed producing the strongest binding affinity to the Mpro of SARS-CoV-2, as compared to positive control (N3 inhibitor). These binding energies were found to be favorable for an efficient docking and resultant. The interaction of the C. ternatea compounds with Mpro active site amino acids is shown in Figures 2 and 3 and summarized in Table 3.
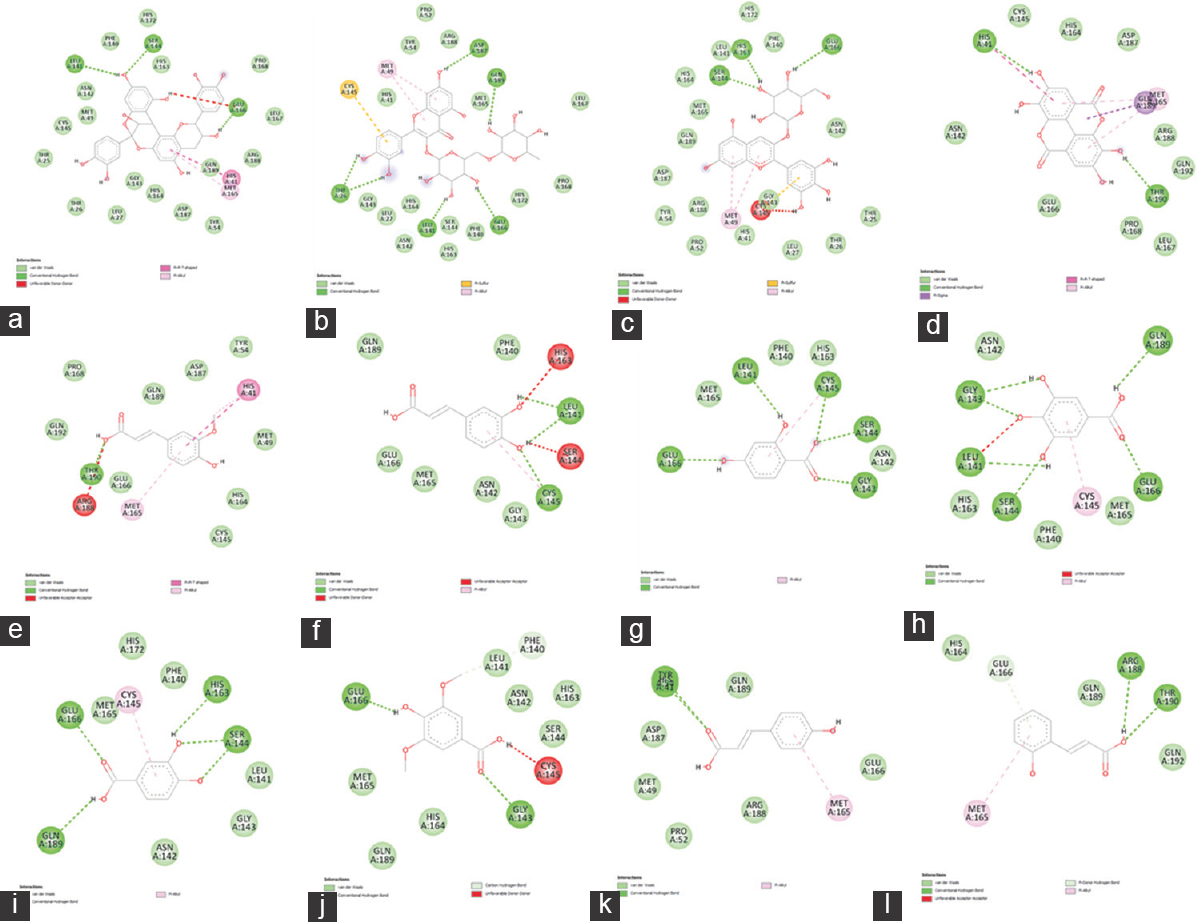
- 2D Diagrams of Clitoria ternatea Compounds Interaction with SARS-CoV-2 Mpro Active Site: (a) Procyanidin A2, (b) Quercetin-3-rutinoside, (c) Delphinidin-3-O-glucoside, (d) Ellagic Acid, (e) Ferulic Acid, (f) Caffeic Acid, (g) 2,4-Dihydroxybenzoic Acid, (h) Gallic Acid, (i)Protocatechuic Acid, (j) Syringic Acid, (k) p-Coumaric Acid, (l) 2-Hydroxycinnamic Acid
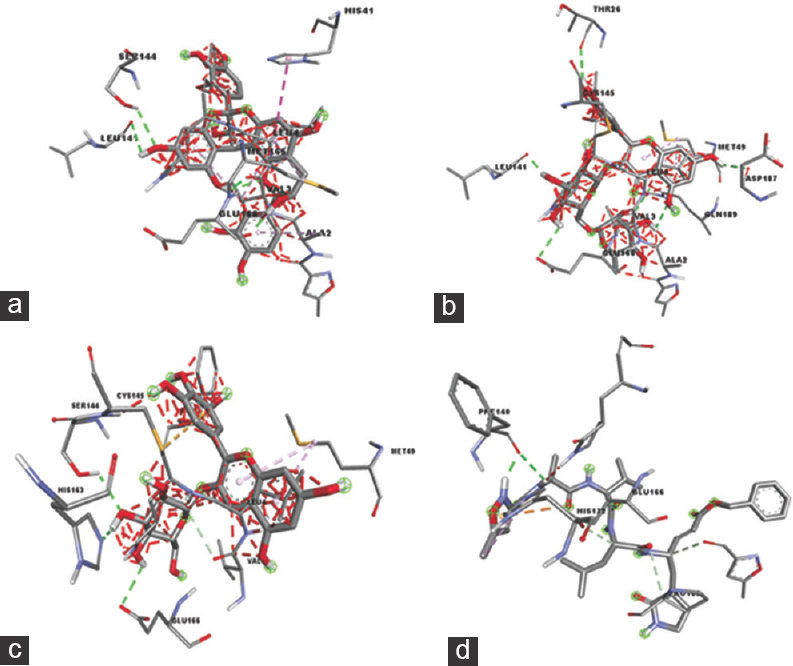
- 3D Diagrams of Clitoria ternatea Compounds Interaction with SARS-CoV-2 Mpro Active Site: (a) Procyanidin A2, (b) Quercetin-3-rutinoside, (c) Delphinidin-3-O-glucoside, (d) N3 inhibitor
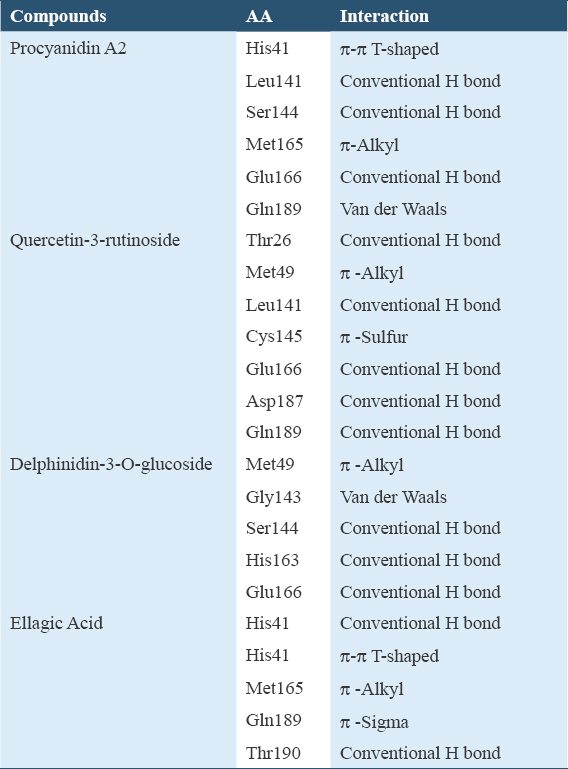
There are two types of hydrogen bonds are detected: Conventional hydrogen bond and carbon hydrogen bond. The carbon-hydrogen bond (CH ··· O) is weaker than the conventional hydrogen bond because CH O’s average distance is longer than the conventional hydrogen bond (NH···O, OH···O, 140 OH···N, and NH···N). However, it is known that the carbon-hydrogen C–H-O bond has an important role in the molecular recognition process.[24,25] The hydrogen bond formation is critical in ligand-protein interaction as it gives a stabilization effect in ligand-protein interaction, which suggests that it has the potential to exhibit potent pharmacological response.[26,27] Besides hydrogen bonds, other key residues interact through hydrophobic bonds (π--Alkyl) and π-sigma and electrostatic bonds (van der Waals). The presence of hydrophobic bonds could be due to any interaction between hydrophobic amino acids with a polar solvent. The π-interaction is believed to give a significant contribution to the ligands’ binding energy to their receptors.[28-30]
The ADMET and drug likeness prediction of the compounds according to Lipinski’s Rule is expressed in Table 4. The hydrogen bond acceptors were checked for not exceeding 10, hydrogen bond donors not exceeding 5, molecular mass not exceeding 500 Da and octanol-water partition co-efficient (log P) not exceeding 5.[31]
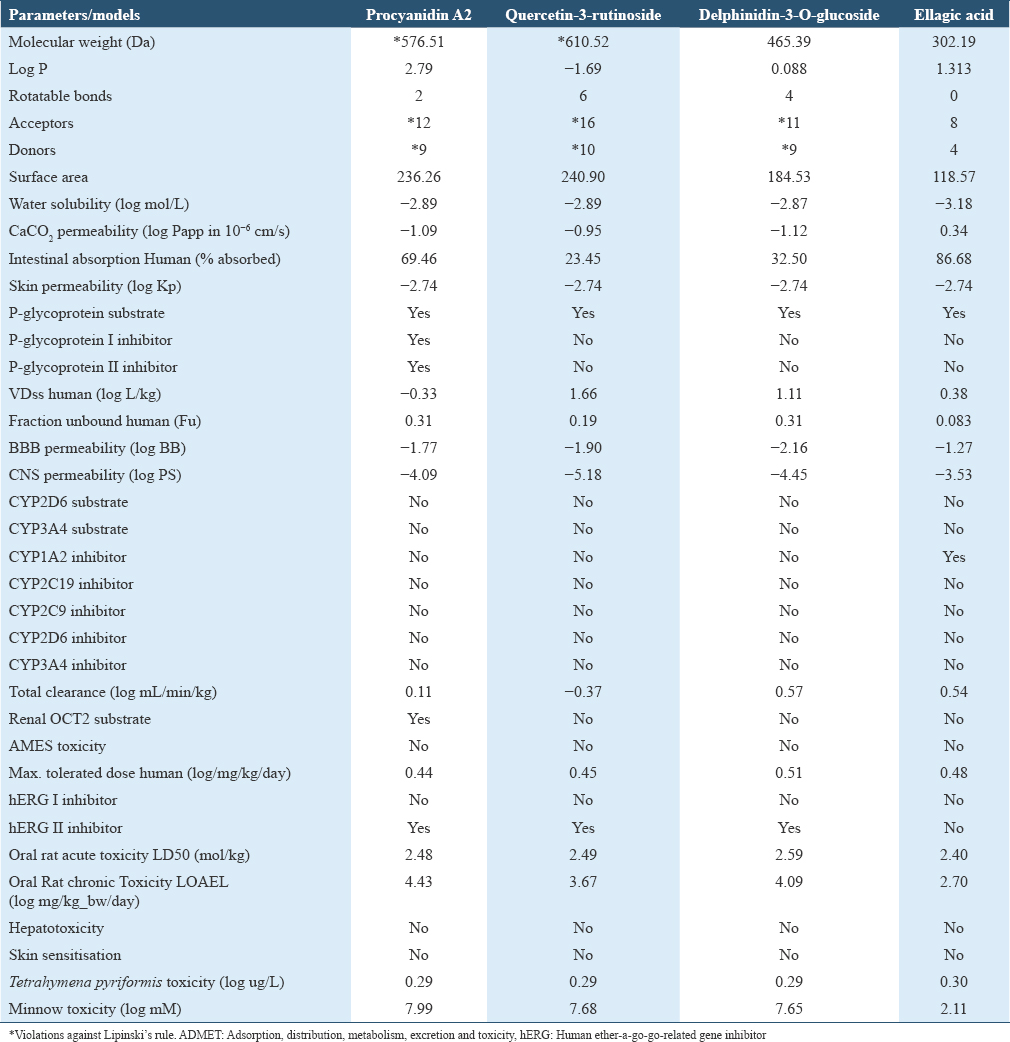
Discussion
Binding affinity is the interaction strength between two or more molecules that bind reversibly to each other.[25] Shityakov and Foerster suggested that most active molecules have binding affinities lower than −6 kcal/moL while inactive molecules show binding affinities higher than −6 kcal/mol.[32] According to a study by Carlson et al., high binding affinity is usually lower than −9 kcal/mol.[33] The positive control, N3 inhibitor shows a binding affinity of −7.5 kcal/mol (≤6 kcal/mol). In this study, the compound with the strongest binding affinity is procyanidin A2 which is −9.3 kcal/mol. It is considered as strong bond against Mpro as the value is lower than −9 kcal/mol. The other compounds that displayed binding affinities lower than −6 kcal/moL including quercetin-3-rutinoside (−8.9 kcal/moL), delphinidin-3-O-glucoside (−8.3 kcal/moL), and ellagic acid (−7.4 kcal/moL). Hence, these four compounds were shown to have strong interaction with Mpro.
The type of molecular interactions including hydrogen (H) bond, hydrophobic, and electrostatic interactions that indicate the ligand docking in favorable conformations with essential amino acid residues are also important.[34,35] The major interactions that contribute to proteins stability and folding configuration equilibria are hydrophobic interactions, greater than H bonds even in the smallest globular proteins.[36,37] H bonds possess strong intermolecular forces between two opposite polarity molecules. This dipole-dipole attraction is crucial for the ligand-receptor bonds.[38] It can also displace protein-bound water molecules into the bulk solvent and therefore enhance ligand binding affinity.[39,40] Besides, electrostatic interactions are related to binding affinity, stability, structure, and chemical characteristics.[34,41,42] It occurs when one atom loses electron to form positive ions and the other atom gains electrons to form negative ions. The orientation of intermolecular structures adsorbed on the wall surface can be verified by electrostatic interactions.[38]
Most of the interactions of the C. ternatea compounds with Mpro active site amino acids are conventional H bonds. To cross the lipid bilayer, a drug should acquire polar interaction with lipophilicity through strong hydrogen bonds.[43] For the compound with the strongest binding affinity, procyanidin A2 consists of three amino acids involved in H bonds (Leu141, Ser144, Glu166), one π-π interaction (His41), one π-Alkyl bond (Met165), and one Van der Waals bond (Gln189). Its greatest stability was contributed by the substantial amount of H bonds as they facilitate protein-ligand binding.[39] The non-covalent π-π stacking interaction is responsible for biological recognition and biomolecular structures organization.[44,45] However, Glu166 also formed an unfavorable donor-donor bond with the receptor Mpro. Unfavorable bonds reduce the stability between the ligand and the receptor. The presence of repulsion forces is indicated by any kind of unfavorable bonds between the two molecules and atom.[46] This suggested that procyanidin A2 may not be suitable to be used as anti-Mpro drug.
Other than procyanidin A2, the compound with binding affinities lower than -6 kcal/mol that displayed unfavorable bonds including delphinidine-3-O-glucoside, which formed the third strongest bond with Mpro is also unsuitable for Mpro inhibitor. Quercetin-3-rutinoside showed binding affinities slightly higher than procyanidin A2 contains five amino acids forming conventional H bonds (Thr26, Leu141, Glu166, Asp187, and Gln189), one π -Alkyl bond (Met49), and one π-Sulfur bond (Cys145). The stability of the protein-ligand complex is high due to the high amount of H bonds, and it has no unfavorable bonds. This result is in link with the findings by Dibha et al. stated that quercetin shows anti-viral effects against Mpro.[43] In addition, although ellagic acid’s binding affinity is somewhat higher than the positive control N3 inhibitor, it also displayed strong stability with Mpro. Conventional H bonds occur at the amino acid sites His41 and Thr190 with one π-π T-shaped bond at His41, one π-Alkyl bond at Met165, and one π-Sigma bond at Gln189. Strong and stable bonds formed on the active sites of Mpro can result in amino acids that inhibit Mpro activation and function.[43]
The pharmacodynamics of the selected molecules in drug research can be analyzed by ADMET and drug likeness prediction. A molecule violating two or more Lipinski’s Rule indicated that it is not orally active.[47] Lipinki’s rule of five (RO5) consists of calculating molecular properties such as log P, polar surface area, number of hydrogen bond donors, number of hydrogen bond acceptors, and molecular weight, which can help assist in predicting the oral action of the respective pharmacological compound.[48] Among the candidate molecules, quercetin-3-rutinoside disobeyed three of the four rules by possessing 610.521 Da molecular weight, 16 hydrogen bond acceptors and 10 hydrogen bond donors. However, Lipinski’s Rule is not the only standard to determine the viability of phytochemicals. The principle is based on relatively simple small molecules and not suitable for complicated natural products.[47] Moreover, the additional hydroxy group and glycoside substituent of quercetin-3-rutinoside contributed to the excessive molecular weight and hydrogen bonds. As quercetin-3-rutinoside is a derivative of quercetin, quercetin was checked for ADMET prediction and found to comply all of the rules.
Besides, the water solubility’s of all candidate molecules are high as they are higher than −6 log moL/L.[49] The polar surface area which is linked to oral absorption or membrane permeability of ellagic acid is <140, proving that it has weak polarity and will be absorbed by the body easier. The other ADMET parameters that indicate absorption properties including the CaCO2 permeability and intestinal absorption of ellagic acid are also better than quercetin-3-rutinoside but both of them have the same skin permeability. The distribution of drugs can be demonstrated by distribution volume (VDss) where log VDss lower than −0.15 is relatively low. Both candidate molecules have log VDss higher than −0.15. Drug metabolism denoted by cytochrome P450 subtypes of the candidate molecules suggested that they can be metabolized by the liver. Nevertheless, the total clearance indicating drug elimination of ellagic acid is greater than quercetin-3-rutinoside.[50,51] The negative Ames toxicity showed no mutagenicity and there is no hepatoxicity, skin sensitization and human ether-a-go-go-related gene inhibitor (hERG) which shows cardiovascular toxicity in all the candidate molecules except hERG II inhibitor in quercetin -3- rutinoside.[47,52] The other toxicity parameters such as LD50 and LOAEL are also in acceptable levels.[53]
One of the limitations of molecular docking study is the confidence of scoring functions providing accurate binding energies are low. It is difficult to predict certain intermolecular interaction precisely such as solvation effects and entropy changes. The scoring functions are also not considering some intermolecular interactions that are showed to contribute in protein-ligand binding affinities such as halogen and guanidine-arginine bonding. Besides, the using of rigid receptor may cause false negatives as its conformation is single and fixed. Constant motion can be shown by a protein between different conformational states with similar energies. However, a protein should be able to acquire many different conformations while binding to different ligands.[54]
Therefore, quercetin-3-rutinoside and ellagic acid are suitable to be used as Mpro inhibitors. Recent in silico studies from Fazadini and Yzzuddin also evidenced that baicalein which is a secondary metabolite of C. ternatea has inhibition effects on Mpro and Nugraha et al. suggested that anthocyanin and ternatin from C. ternatea are potential to be used for COVID-19 oral manifestation therapy.[38,55] As the results in this study shows great binding affinities of C. ternatea’ bioactive compounds against Mpro, further in vitro and in vivo research on using C. ternatea as potential Mpro antagonist should be carried out to provide more incontrovertible evidence.
Conclusion
In this study, two bioactive compounds of C. ternatea, quercetin-3-rutinoside and ellagic acid showed strong binding affinities and stability against Mpro, indicating that they are potential in inhibiting SARS-CoV-2. Hence, this natural product is suitable to be used as an antiviral drug.
Authors’ Declaration Statements
Ethics approval and consent to participate
The study is exempt from review by the Taylor’s University Human Ethics Committee (TUHEC) based on the basis that this type of study is non-human subject research and waived the need for informed consent.
Availability of data and material
The data that support the findings of this study are available from the corresponding author upon reasonable request.
Competing interests
The authors declare that there is no conflict of interest.
Funding Statement
This research was funded by the Ministry of Higher Education (MoHE) Malaysia through Fundamental Research Grant Scheme (FRGS/1/2020/SKK0/TAYLOR/02/2). The funding for niche area research was supported by the National Cancer Council Malaysia (MAKNA) Cancer Research Award (CRA) 2021 (MAKNA/2021/SBS/001). This material is based upon work supported by the Malaysia Toray Science Foundation (MTSF/2021/SBS/001).
Authors’ Contributions
YQT conceived the study idea. CYC and SXYK completed the bioinformatics analysis and drafted the manuscript with contributions from all authors. AYYC and YQT reviewed and supervised the work. All authors contributed to the article and the final version of the manuscript was reviewed and approved by all the authors.
References
- 2019 novel coronavirus (2019-nCoV) outbreak:A new challenge. J Glob Antimicrob Resist. 2020;21:22-7.
- [Google Scholar]
- Detection of anti-SARS-CoV-2 antibodies and its seroprevalence in Zavidovići municipality of Zenica-Doboj Canton, Bosnia and Herzegovina. Int J Health Sci (Qassim). 2022;16:3-8.
- [Google Scholar]
- SARS CoV-2 spike protein in silico interaction With ACE2 receptors from wild and domestic species. Front Genet. 2021;12:571707.
- [Google Scholar]
- SARS-CoV-2:Structure, biology, and structure-based therapeutics development. Front Cell Infect Microbiol. 2020;10:587269.
- [Google Scholar]
- Computational screening of antagonists against the SARS-CoV-2 (COVID-19) coronavirus by molecular docking. Int J Antimicrob Agents. 2020;56:106012.
- [Google Scholar]
- Potential bioactive glycosylated flavonoids as SARS-CoV-2 main protease inhibitors:A molecular docking and simulation studies. PLoS One. 2020;15:e0240653.
- [Google Scholar]
- In silico analysis of SARS-CoV-2 proteins as targets for clinically available drugs. Sci Rep. 2022;12:5320.
- [Google Scholar]
- An integrated in silico immuno-genetic analytical platform provides insights into COVID-19 serological and vaccine targets. Genome Med. 2021;13:4.
- [Google Scholar]
- Prospects of antiviral drugs derived from natural products:Targeting SARS-CoV entry and replication. Curr Tradit Med. 2021;7:3-9.
- [Google Scholar]
- Molecular docking and dynamics simulation revealed the potential inhibitory activity of ACEIs against SARS-CoV-2 targeting the hACE2 receptor. Front Chem. 2021;9:661230.
- [Google Scholar]
- DeepDTA:Deep drug-target binding affinity prediction. Bioinformatics. 2018;34:i821-9.
- [Google Scholar]
- A potential of Telang tree (Clitoria ternatea) in human health. Food Res. 2018;2:415-20.
- [Google Scholar]
- Clitoria ternatea L. Petal bioactive compounds display antioxidant, antihemolytic and antihypertensive effects, inhibit a-amylase and a-glucosidase activities and reduce human LDL cholesterol and DNA induced oxidation. Food Res Int. 2020;128:108763.
- [Google Scholar]
- The ayurvedic medicine Clitoria ternatea-from traditional use to scientific assessment. J Ethnopharmacol. 2008;120:291-301.
- [Google Scholar]
- Phytochemistry and pharmacological activities of Clitoria ternatea. Int J Nat Soc Sci. 2017;4:1-10.
- [Google Scholar]
- In silico study:The blue butterfly pea flower (Clitoria ternatea L.) compound has potential for herbal medicine for COVID-19. World J Pharm Res. 2022;11:970-85.
- [Google Scholar]
- Synthesis, characterization and antimicrobial activity of selenium nanoparticles with Clitoria ternatea on oral pathogens. Int J Health Sci (Qassim). 2022;6:2529-38.
- [Google Scholar]
- Design of wide-spectrum inhibitors targeting coronavirus main proteases. PLoS Biol. 2005;3:e324.
- [Google Scholar]
- The newly emerged SARS-like coronavirus HCoV-EMC also has an “Achilles'heel”:Current effective inhibitor targeting a 3C-like protease. Protein Cell. 2013;4:248-50.
- [Google Scholar]
- Structure of main protease from human coronavirus NL63:Insights for wide spectrum anti-coronavirus drug design. Sci Rep. 2016;6:22677.
- [Google Scholar]
- Structure of Mpro from SARS-CoV-2 and discovery of its inhibitors. Nature. 2020;582:289-93.
- [Google Scholar]
- A systematic analysis of atomic protein-ligand interactions in the PDB. Medchemcomm. 2017;8:1970-81.
- [Google Scholar]
- Antimicrobial screening to molecular docking of newly synthesized ferrocenyl-substituted pyrazole. Int J Health Sci (Qassim). 2022;16:3-12.
- [Google Scholar]
- Pharmacokinetic and molecular docking studies to design antimalarial compounds targeting Actin I. Int J Health Sci (Qassim). 2021;15:4-15.
- [Google Scholar]
- Cation-p interactions in protein-ligand binding:Theory and data-mining reveal different roles for lysine and arginine. Chem Sci. 2018;9:2655-65.
- [Google Scholar]
- In silico investigation of potential inhibitors to main protease and spike protein of SARS-CoV-2 in propolis. Biochem Biophys Rep. 2021;26:100969.
- [Google Scholar]
- A molecular docking study of SARS-CoV-2 main protease against phytochemicals of Boerhavia diffusa Linn. For novel COVID-19 drug discovery. Virusdisease. 2021;32:46-54.
- [Google Scholar]
- In silico predictive model to determine vector-mediated transport properties for the blood-brain barrier choline transporter. Adv Appl Bioinform Chem. 2014;7:23-36.
- [Google Scholar]
- Differences between high- and low-affinity complexes of enzymes and nonenzymes. J Med Chem. 2008;51:6432-41.
- [Google Scholar]
- In silico analysis of molecular interactions between the anti-apoptotic protein survivin and dentatin, nordentatin, and quercetin. J Phys Conf Ser. 2018;1073:032001.
- [Google Scholar]
- Contribution of hydrophobic interactions to protein stability. J Mol Biol. 2011;408:514-28.
- [Google Scholar]
- Competition of electrostatic and hydrophobic interactions between small hydrophobes and model enclosures. J Phys Chem B. 2010;114:7294-301.
- [Google Scholar]
- Electrostatic potential energy in protein-drug complexes. Curr Med Chem. 2021;28:4954-71.
- [Google Scholar]
- Regulation of protein-ligand binding affinity by hydrogen bond pairing. Sci Adv. 2016;2:e1501240.
- [Google Scholar]
- Electrostatic interactions in protein structure, folding, binding, and condensation. Chem Rev. 2018;118:1691-741.
- [Google Scholar]
- Computational screening of repurposed drugs targeting Sars-Cov-2 main protease by molecular docking. Sudan J Med Sci. 2022;17:387-400.
- [Google Scholar]
- Biological activity of kencur (Kaempferia galanga L.) against SARS-CoV-2 main protease:In silico study. Int J Health Sci. 2022;6:468-80.
- [Google Scholar]
- Aromatic interactions at the ligand-protein interface:Implications for the development of docking scoring functions. Chem Biol Drug Des. 2018;91:380-90.
- [Google Scholar]
- Comparative in silico molecular docking analysis of L-threonine-3-dehydrogenase, a protein target against African trypanosomiasis using selected phytochemicals. J Appl Biotechnol Rep. 2019;6:101-8.
- [Google Scholar]
- ADMET-score-a comprehensive scoring function for evaluation of chemical drug-likeness. Medchemcomm. 2019;10:148-57.
- [Google Scholar]
- In silico identification of potential inhibitors from rumex vesicarius against DPP4 of diabetes mellitus. Int J Health Sci (Qassim). 2022;6:1002-17.
- [Google Scholar]
- Molecular docking studies of some selected Gallic acid derivatives against five non-structural proteins of novel coronavirus. J Genet Eng Biotechnol. 2021;19:16.
- [Google Scholar]
- In silico ADME and toxicity prediction of ceftazidime and its impurities. Front Pharmacol. 2019;10:434.
- [Google Scholar]
- Animal venom-derived antimicrobial peptides:Novel and improved weapon for cancer treatment. Malays J Biochem Mol Biol. 2020;1:90-8.
- [Google Scholar]
- hERG toxicity assessment:Useful guidelines for drug design. Eur J Med Chem. 2020;195:112290.
- [Google Scholar]
- Computational analysis of calculated physicochemical and ADMET properties of protein-protein interaction inhibitors. Sci Rep. 2017;7:46277.
- [Google Scholar]
- Molecular docking:A powerful approach for structure-based drug discovery. Curr Comput Aided Drug Des. 2011;7:146-57.
- [Google Scholar]
- Molecular docking of anthocyanins and ternatin in Clitoria ternatea as coronavirus disease oral manifestation therapy. J Adv Pharm Technol Res. 2021;12:362-7.
- [Google Scholar]