Translate this page into:
Detection of Novel hub-methylated differentially expressed genes in pregnant women with gestational diabetes mellitus via WGCNA of epigenome-wide and transcriptome-wide profiling
Address for correspondence: Hamdan Z. Hamdan, Department of Pathology, College of Medicine, Qassim University, Buraidah, Saudi Arabia. Phone: +966 565471080. E-mail: h.abualbasher@qu.edu.sa
This is an open-access article distributed under the terms of the Creative Commons Attribution-Noncommercial-Share Alike 3.0 Unported, which permits unrestricted use, distribution, and reproduction in any medium, provided the original work is properly cited.
This article was originally published by Qassim Uninversity and was migrated to Scientific Scholar after the change of Publisher.
Abstract
ABSTRACT
Objectives:
Gestational diabetes mellitus (GDM) is a prevalent metabolic disorder that adversely affects pregnant women and their growing fetuses. Evidence suggests that genetic and epigenetic modifications, such as DNA methylation, may contribute to the disease phenotype. This study aimed to identify GDM-related hub-methylated genes involved in GDM pathogenesis.
Methods:
RNA-seq transcriptomic-wide data (GSE203346) and microarray epigenomic-wide data (GSE106099) were obtained from the Gene Expression Omnibus. Weighted Gene Co-expression Network Analysis (WGCNA) and differential gene expression (DEGs) analysis were performed on the RNA-seq data using the “R” packages “WGCNA” and “DESeq2,” respectively. Differentially methylated genes (DMGs) were identified using the “limma” package.
Results:
WGCNA identified 18 modules, with only two modules [MEyellow r = −0.32; P = 0.042 and MEmagenta r = −0.32; P = 0.041] showing significant inverse correlations with GDM and one module [MEblue r = 0.35; P = 0.026], showing a direct correlation. Following intersecting the hub genes from WGCNA, DEGs and DMGs, six hub genes were identified as hypomethylated and highly expressed (UCKL1, SHANK2, GDPD5, CMYA5, ESRRG, NOS3), while two genes (DPYSL3 and FTH1) were hypermethylated and showed low expression. Gene set enrichment analysis revealed that the GDM-related hub DMGs were mainly enriched in pathways related to ferroptosis, VEGF signaling, and arginine and proline metabolism.
Conclusion:
This multi-omics study identified eight novel GDM-related hub DMGs in placental tissue from GDM cases, suggesting their potential involvement in GDM pathogenesis. Further study is needed.
Keywords
DNA-methylation
epigenetics
gene expression
gestational diabetes mellitus
RNA-seq
weighted gene co-expression network analysis
Introduction
Gestational diabetes mellitus (GDM) is a common medical disorder that affects 15.8% of pregnant women worldwide.[1] It is characterized by hyperglycemia that commenced during the first or second trimester of the pregnancy.[2] GDM is associated with numerous maternal and fetal adverse events, including hypertensive-related conditions such as preeclampsia, preterm delivery, fetal macrosomia, and delivery-associated complications like shoulder dystocia.[3] After birth and removal of the placenta, blood glucose levels typically return to normal in most cases, yet mothers remain at an increased risk of developing Type 2 diabetes mellitus and their offsprings are prone to have metabolic syndrome and cardiovascular complication later in their life.[4] Therefore, efforts have been made to early detect and manage GDM to mitigate the adverse events that may arise during or after pregnancy for the mother and her fetus.[5,6]
The exact cause of GDM remains unclear, however, multiple risk factors such as maternal obesity, family history of diabetes, previous history of GDM, ethnicity, and genetic factors have been identified.[7] Besides these factors, epigenetics modifications like DNA methylation have been mooted also as a contributing factor for the GDM development.[8] DNA methylation is known to downregulate gene expression without altering the DNA sequence. In contrast, hypomethylation is associated with the upregulation of gene expression. Evidence showed that placental and fetal cord DNA methylation of certain genes, such as AHRR and PTPRN2, is linked to maternal hyperglycemia and GDM.[9] On the other hand, hypomethylation of the CYP2E1 gene, which results in its upregulation, has also been associated with GDM development.[10] Previous studies have reported differentially methylated and transcriptomic genes in GDM compared to non-GDM women’s placenta tissues.[11,12] Yet, previous studies applied methods like DNA methylation chips or high throughput methylation and transcriptome studies usually focused on the individual methylated regions in genes without focusing more on the overall expressional pattern.[9,13]
Weighted gene co-expression network analysis (WGCNA) is a bioinformatics technique employed to identify patterns of genes that are either expressed or suppressed together in response to a specific condition and to correlate the expression of these genes with a specific trait. This technique allows researchers to identify sets of genes that work in concert, rather than studying a single gene expression[]14] By this means, we can identify possible transcriptomic and methylation hub genes for GDM that could be used either as a diagnostic marker or potential therapeutic targets.
Dearth studies investigated both the transcriptomic and epigenomic signature in GDM.[15,16] Most of these studies used different approaches to investigate GDM expressions, hence reported different hub genes. In this study, we applied multi-omics approach that combines transcriptomic and epigenomics data to explore the hub genes related to GDM. Identification of hypermethylated genes in GDM can be confirmed by assessing its transcription profile by RNA-seq, in such way both sides _ transcriptional and epigenetics_ of expressional alteration can be assessed. The findings of this study add to the current GDM literature and deepening our understanding of the GDM transcriptomic and epigenetics knowledge. Moreover, identified hub genes can help in designing appropriate epigenetics-targeted drugs against these hub genes or used them as a potential diagnostic biomarker. Therefore, this study is worthwhile.
Methods
Datasets
The Gene Expression Omnibus database was used to search and download datasets that investigated the gene expression and DNA methylation profile of placental tissues extracted from women with GDM and compared it with healthy parturient controls. The dataset (GSE203346), which includes RNA-sequencing profiles of placental tissues that extracted from 21 pregnant women with GDM and 20 pregnant women without GDM is used to determine the differentially expressed genes (DEGs) between the cases of GDM and healthy controls and constructing the weighted gene co-expression network. The dataset (GSE106099) contains DNA methylation profiles that obtained from placental extracts from 12 GDM pregnant women and compared with 18 healthy controls parturient women to obtain the differentially methylated genes (DMGs). The DNA methylation analysis was done using Illumina HumanMethylation450 BeadChip platform, Table 1. The flow-chart shown in Figure 1 shows the steps that were followed in this study.
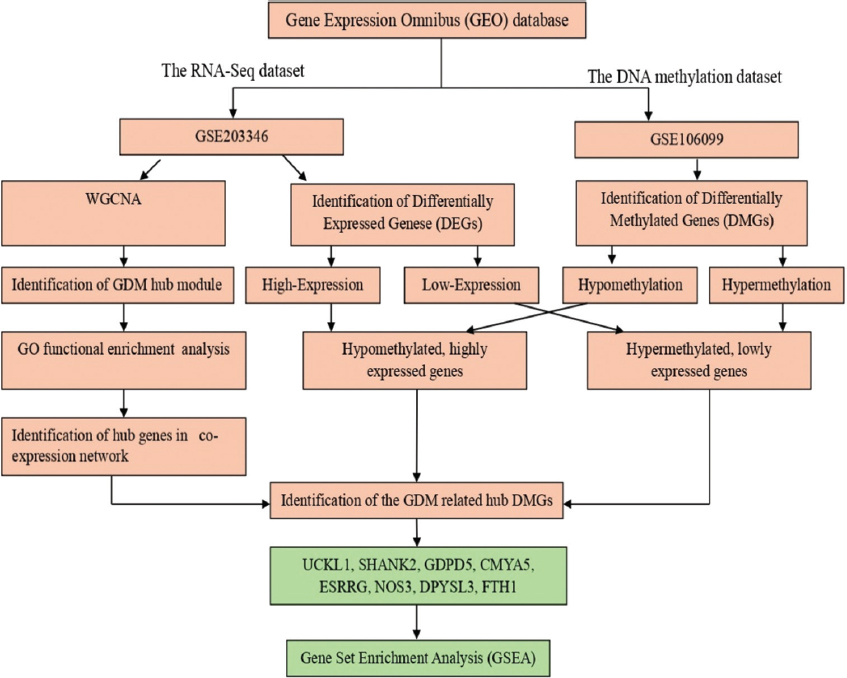
- Study flowchart

Construction of weighted gene co-expression network
To identify the combined set of genes that are co-expressed or co-suppressed together in the case of GDM, the Weighted Gene Expression Network Analysis was constructed using the R package “WGCNA.” Very briefly, the genes with low expression in more than 75% of the samples were removed. Furthermore, samples which are flagged as outliers are removed prior analysis. The minimum mean connectivity between the modules is guaranteed after performing scale-free topology index (R2) and calculating the soft threshold power of 7. Adjacency matrix is calculated and converted to topological overlap matrix that is used to minimize the noises. The expression of each module is expressed by constructing module eigengene (MEs) and expressing the correlation coefficient with a probability value.
DEG analysis
RNA-sequencing dataset was processed and analyzed using the statistical software R 4.3.2 through the interface RStudio. The package “DESeq2” was used to identify the DEGs. Since this is an exploratory study aiming to capture a wide range of potential candidate genes with significant alterations in expression, genes with |log2fold-change| >0.5 and P < 0.05 were considered as DEGs as described in a previous study.[17]
Identification of hub modules and hub GDM genes
Following obtaining of the MEs, Pearson correlation score is obtained to evaluate the genes related to each module and expressed as gene module membership. Moreover, a Pearson correlation analysis is performed to correlate between the genes and GDM as clinical trait. Cytoscape software was used with ClueGo plug-in to perform functional cluster analysis for the significant modules related to GDM. To systematically approach the hub genes, first the modules selected if it was significantly correlated with GDM. Then the intra-module hub genes were identified if the Pearson correlation for the gene module membership (|cor.| >0.8) and the Pearson correlation for the relationship to GDM (|cor. Gene Trait Significance| >0.2) for a gene.
DNA methylation analysis and identification of GDM-related hub-methylated genes
To identify the DMGs between GDM cases and healthy controls, the DNA methylation dataset (GSE106099) was analyzed using R packages “limma.” A gene was defined as DMG if (|log2FC| >2.0) with a P < 0.05. Accordingly, the genes were labeled as hypomethylated (high-expression) or hypermethylated (low-expression) genes. The identified hypo and hyper methylated genes were overlapped with the GDM-DGE and hub genes in GDM modules to identify GDM-related hub DMGs. Moreover, factors such as maternal age, gestational age, and body mass index were comparable between the two groups (GDM vs. controls) in the original dataset.[18] Otherwise, and to eliminate any possible confounding effect of these factors, they were included as a covariate in the limma package during the analysis.
Gene set enrichment analysis
The R package “ClusterProfiler” was used to perform the gene enrichment analysis. The analysis used the Kyoto Encyclopedia of Genes and Genomes pathway in addition to Gene Ontology (GO) database to identify possible mechanisms for the differential expressed genes explaining the pathogenicity of gestational diabetes. GO database reports gene functions in three categories; biological processes, cellular components, and molecular functions.
Results
WGCNA result
Following samples clustering, all 41 samples were included in the WGCNA [Figure 2a]. The threshold power to detect the significant minimum mean connectivity and ensure scale-free topology was set at power = 7 [Figure 2b]. A total of 18 modules were generated with three modules that had significant correlations with GDM [Figure 2c]. Module MEblue with a total of 1,470 genes had a significant positive correlation with GDM (r = 0.35; P = 0.026) while modules MEyellow 641 genes (r = −0.32; P = 0.042) and MEmagenta 82 genes (r = −0.32; P = 0.041) had significant negative correlation with GDM [Figure 2d].
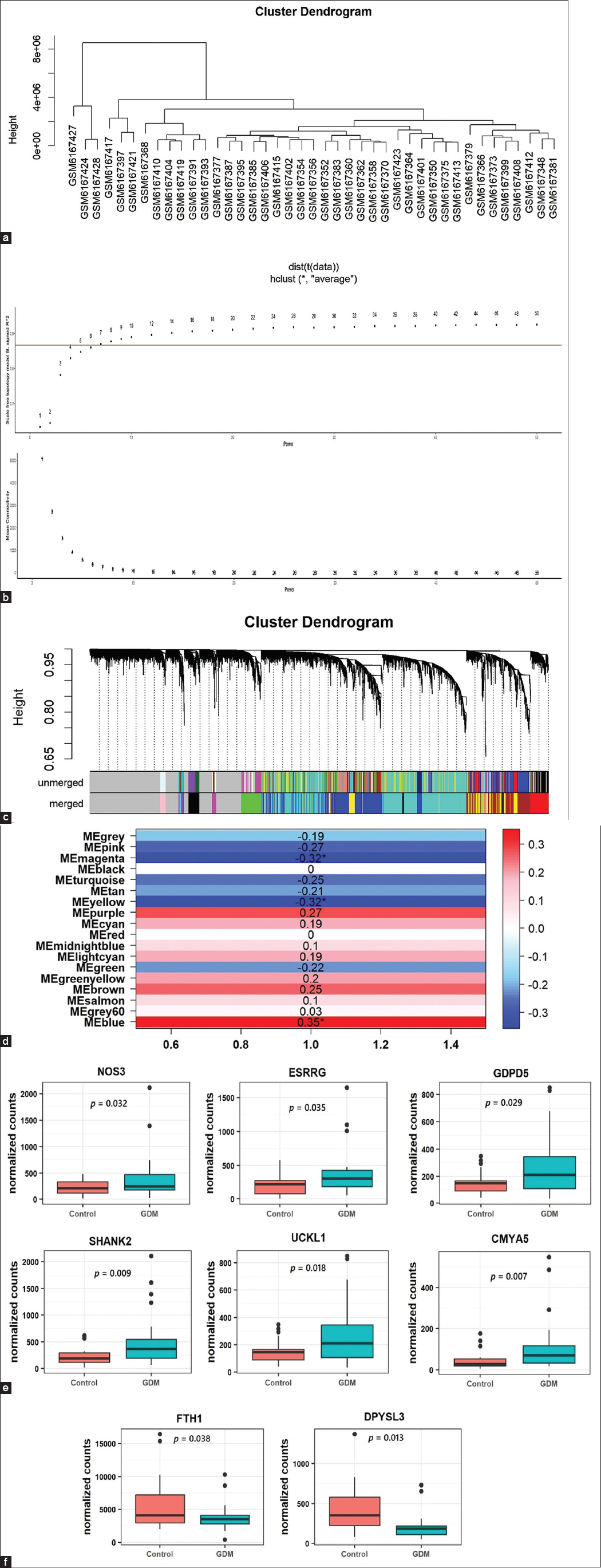
- (a) Cluster dendrogram for 41 samples. (b) Showing the screening for soft threshold power for WGCNA. Above: scale-free fit index analysis for different power thresholds. Below: Calculation of mean connectivity corresponding for the different threshold power. (c) Cluster dendrogram of all expressed genes. (d) Module-disease correlation heatmap. (e) Relative expression levels of UCKL1, SHANK2, GDPD5, CMYA5, ESRRG, and NOS3 genes were significantly increased in GDM cases compared to controls. (f) Relative expression levels of DPYSL3 and FTH1genes were significantly lower in GDM cases compared to controls
The gene enrichment analysis for the blue module was enriched mainly for terms related to regulation of gene expression (histone lysine methylation, spliceosomal complex formation, regulation of histone modification, regulation of transcription elongation), Figure 3a. While, the yellow module genes enriched mainly with glycolysis/gluconeogenesis, oxidoreductase activity, mineral absorption, and others, Figure 3b. For magenta module, it is enriched only under the term LAIR2 binds collagen, Figure 3c.
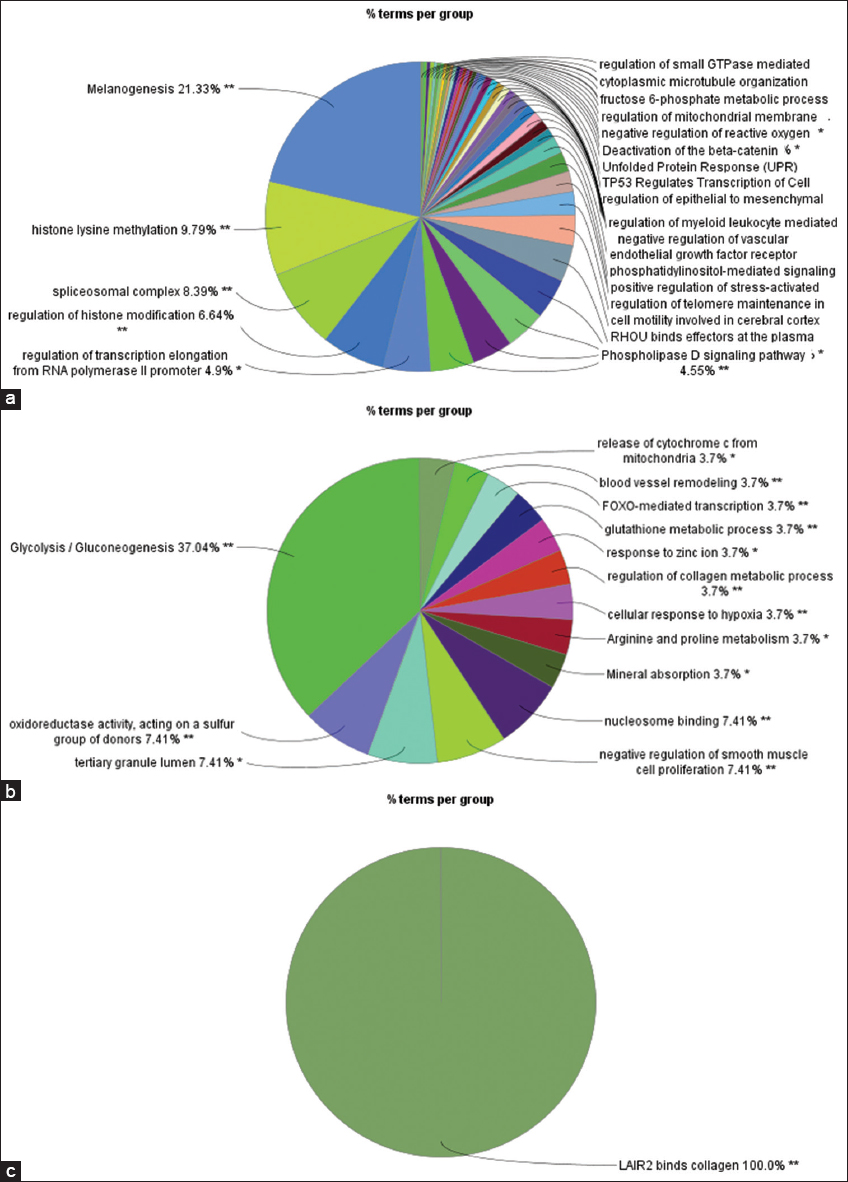
- (a) GO Enrichment analysis of genes in blue module. (b) GO Enrichment analysis of genes in yellow module. (c) GO Enrichment analysis of genes in magenta module. GO: Gene ontology
Identification of hub genes
Co-expression analysis in MEblue module showed that 260 genes were highly correlated with the module blue membership and 828 genes were related to GDM. The overlapped genes were 215 within the MEblue module. For the MEyellow module, a total of 108 genes were found to be highly connected within the module and 356 genes were found to be related to GDM. Eighty-two genes were found to be potential hub genes in MEyellow module. MEmagenta module showed that 12 genes were highly connected to the module and 51 genes were related to GDM. Only 11 genes were potentially hub genes in MEmagenta module.
Identification of GDM-related hub DMGs
In the expression profile of RNA-seq dataset (GSE203346), a total of 83 genes were found to be differentially downregulated and 310 genes were upregulated in GDM samples compared with healthy control placental samples. With regards to (GSE106099) dataset, which contains the methylation data, a total of 6,053 genes were found hypermethylated while 4,716 were hypomethylated. The GDM-related DMGs were identified by comparing the DMGs with DEGs. The GDM-related hub DMGs were identified by overlapping the co-expression hub genes and the GDM-related DMGs, and 6 genes (UCKL1, SHANK2, GDPD5, CMYA5, ESRRG, NOS3) were identified as hypomethylated-high expression [Figure 2e] and two genes (DPYSL3, FTH1) as hypermethylated-low expression genes [Tables 2 and 3, Figure 2f].
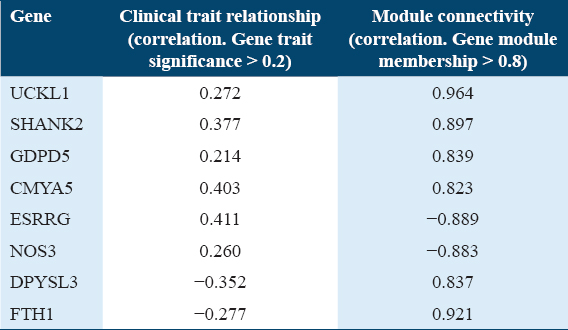
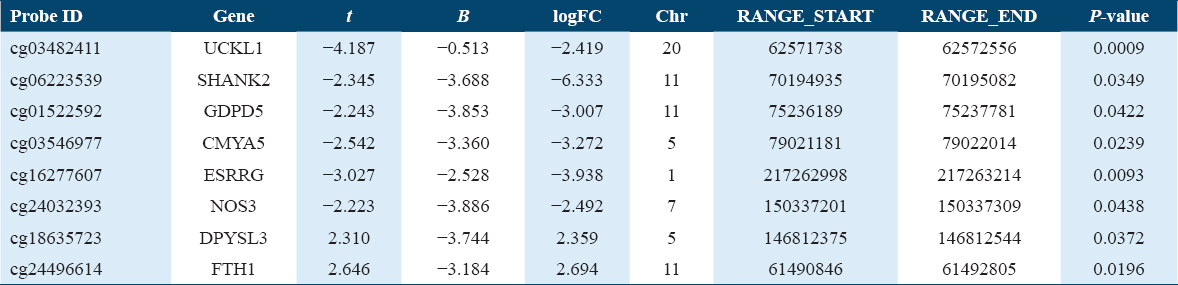
Gene set enrichment analysis
The UCKL1 gene is enriched among the main genes involved in the pyrimidine, nucleotide, and drug metabolism pathways [Figure 4a].
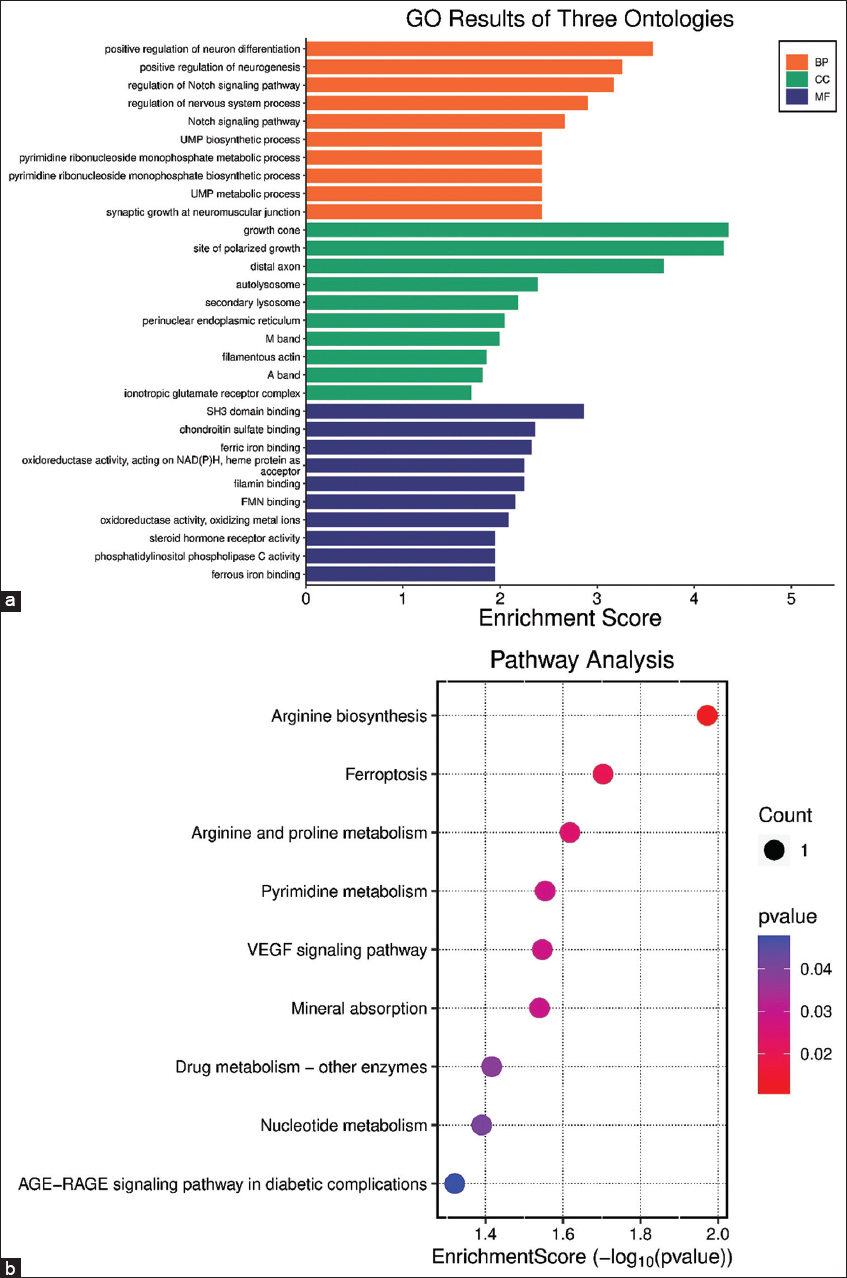
- (a) Gene set Enrichment Analysis scores in BP: Biological process; CC: Cellular component; MF: Molecular function for the UCKL1, NOS3, and FTH1 hub genes. (b) Kyoto Encyclopedia of Genes and Genomes pathways enrichment scores for the UCKL1, NOS3, and FTH1 hub genes
While NOS3 gene is enriched for the genes related to metabolism of Arginine and proline amino acids. Furthermore, it is involved in the VEGF signaling pathway and AGE-RAGE signaling pathway in diabetic complications [Figure 4b]. FTH1 genes contributed to the pathway of ferroptosis and mineral absorption, Table 4.
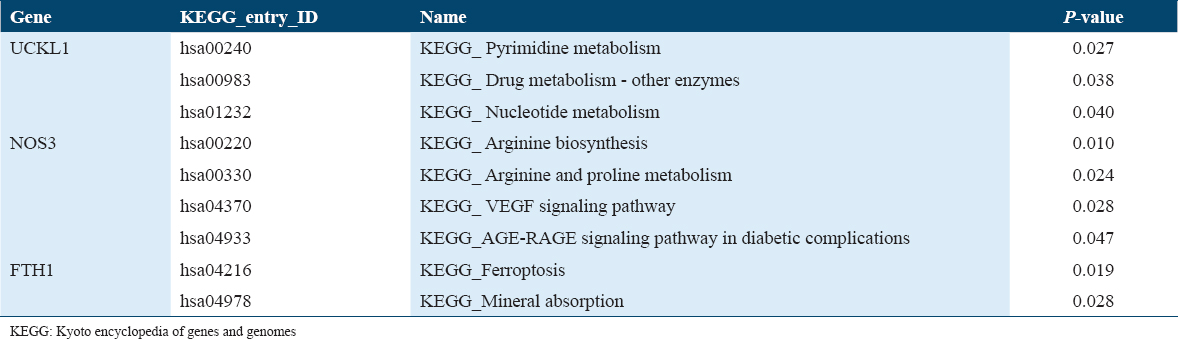
Discussion
Although GDM has been studied for ages, its exact cause remains unclear. A growing body of evidence pointed to the involvement of genetic and epigenetic factors in GDM pathogenesis.[19,20] It has been observed that offspring of mothers with GDM are more likely to develop metabolic and cardiovascular complications later in life, with female offspring particularly at risk of developing GDM themselves. This cycle, known as transgenerational cycle is attributed to epigenetic changes inherited form GDM mother.[21] These epigenetic modifications are passed down with DNA without changing the DNA sequences.[22]
WGCNA is a bioinformatics tool that allows the identification of the genes that are co-expressed or co-repressed together in relation to specific trait.[14] In this study, WGCNA was employed to identify hub genes associated with GDM. Three modules were identified in this study that is associated significantly with GDM trait. The blue module, containing 1,470 genes, was positively correlated with GDM, while the yellow and magenta modules containing 641 and 82 genes, respectively, were negatively correlated. Genes in the blue module were enriched for histone modifications, melanogenesis, signaling transduction molecules, and reactive oxygen species (ROS) regulation. Histone modifications involved histone acetylation and methylation to the histone lysine residues. However, epigenetics studies focused more on DNA methylation, and little is known about the effects of histone modifications in GDM.[23] However, acetylation of histone-3 (H3) is associated with activation of gene transcription as it allows the gene template to be accessible by the transcription machinery.[24] Hepp et al. reported a significant pan down-regulation of H3 acetylation in placenta isolated from GDM patients.[25] Deacetylation of H3 is catalyzed by a family of deacetylase enzymes, which render downregulation of gene expression. Both, histone deacetylase-5 (HDAC5) and HDAC4 were highly expressed among the blue module genes in this study, which is align with Hepp et al. finding.[25] The yellow module genes were enriched for glucose metabolism, zinc ion response and minerals absorption. The relationship between minerals absorption, ROS and GDM is discussed in the context of oxidative stress. Many antioxidative enzymes, such as glutathione peroxidase, catalase, and superoxide dismutase, are metalloenzymes that require selenium, zinc, or copper for optimal activity.[26] Disturbances in serum levels of these metals (selenium, zinc, copper, and others) have been linked to GDM, along with evidence of oxidative stress.[27,28] Selenium supplementation has been shown to have beneficial effects on blood glucose levels, while supplementation with zinc, calcium, and magnesium has improved oxidative stress biomarkers and reduced the incidence of macrosomia in newborns.[29,30] In the magenta module, genes related to LAIR2 (leukocyte-associated immunoglobulin-like receptor 2) were enriched, which binds to collagen. LAIR2 is highly expressed in extravillous trophoblasts, which invade the maternal decidua during early implantation.[31] LAIR2 and its homolog LAIR1 compete for collagen ligand receptors in leukocytes, modulating the immune response.[32,33] One hypothesis regarding GDM pathogenesis involves the unique adaptation of the maternal immune system to the placenta and growing fetus.[34] Dysregulation of the immune system in this context is associated with immune cell activation and altered cellular and humoral immune responses.[35] Little is known about the specific role of LAIR2 in GDM, but it is possible that abnormal modulation of the immune response by LAIR2 contributes to the diabetogenic process of GDM.
The multi-omic analysis in this study identified 8 total GDM-related hub DMGs genes including six hypomethylated-high expression genes (UCKL1, SHANK2, GDPD5, CMYA5, ESRRG, and NOS3) and two hypermethylated-low expression genes (DPYSL3 and FTH1).
Ferroptosis pathway is a form of non-apoptotic cell death pathway, is triggered by cellular iron overload, which primarily increases ROS production and oxidative stress. This results in extensive lipid peroxidation and cellular damage, including damage to pancreatic β-cells.[36,37] A growing body of evidence links ferroptosis to the GDM development.[38,39] At the molecular level, ferroptosis pathway is suppressed by SLC7A11, which is upregulated by a transcription factor Nrf2. UCKL1 a hypomethylated-high expression GDM-related hub gene identified in this study, has been shown to upregulate the Nrf2. In other words, UCKL1 overexpression appears protective against ferroptosis and seems responsive to GDM.[40] On the other hand, FTH1 gene, which is identified hypermethylated-low expression gene in this study, is also related to ferroptosis pathway. FTH1 gene encodes the ferritin heavy chain-1 protein, which is involved in regulating and storing the intracellular iron. A study by Tian et al., reported that overexpression of FTH1 gene inhibits ferroptosis pathway not only by storing excess iron but also by activating ferritinophagy, a distinct mechanism of autophagy specific to manage ferritin overaccumulation and mitigates ferroptosis-induced damage.[41] The finding of this study is consistent with findings by Dang et al., who reported that FTH1 downregulation promotes ferroptosis in astrocytes.[42] That is to say, upregulation of UCKL1 is protective against ferroptosis, insulin resistance and GDM, while FTH1 downregulation may exacerbate ferroptosis, insulin resistance and precipitate GDM. Notably, drugs such as resveratrol[43] and fucoxanthin,[44] are targeting and inhibiting ferroptosis pathway by up-regulating FTH1, SLC7A11 and Nrf2. Given the role of ferroptosis pathway in GDM pathogenesis, these drugs may be of benefit for GDM patients, yet their exact role worth future investigations.
CMYA5, which encodes cardiomyopathy-associated protein 5, is expressed mainly in muscle tissue, including cardiac muscle. Variants of CMYA5 have been associated with hypertrophic cardiomyopathy.[45,46] Offspring of mothers with GDM are at increased risk of developing hypertrophic cardiomyopathy as a complication of GDM.[47,48] Moreover, CARDIA study has concluded that GDM is directly involved in left heart hypertrophy and systolic dysfunction in young adults.[49] In animal models, the study also observed an increased incidence of cardiac hypertrophy cases in offspring of diabetic rats more frequently than non-diabetic controls.[50] Furthermore, a recent study by Chen et al. also found that four GDM-related hub genes were enriched in terms related to cardiac muscle functions.[15] Pathologically, there is no direct link has been established between CMYA5 and GDM, this gene may help explain the cardiac dysfunction observed in offspring of GDM mothers.
NOS3, which encodes the endothelial form of nitric oxide (NO) synthase enzyme, was another hypomethylated and overexpressed hub gene in this study. NO is a vasodilator agent that is increased in response to placental vascular dysfunction. The association between fetoplacental dysfunction and GDM is well-documented.[51,52] This fetoplacental dysfunction in GDM is associated with hyperglycemia and hyperinsulinemia, the hallmarks of insulin resistance.[53-55] Previously, NOS3 gene has been implicated in the insulin resistance and pathogenesis of GDM.[56,57] It is important to note that overproduction of NO can lead to the formation of its free radical form, peroxynitrite, which can directly damage pancreatic tissues and endothelial cells. This exacerbates hyperglycemia and contributes to fetoplacental dysfunction.[55] In addition, pregnancy itself is a state of insulin resistance,[58] where mediators like TNF-α are significantly elevated, particularly in obese individuals. TNF-α plays a role in destabilizing NOS3 mRNA, reducing the likelihood that transcribed NOS3 mRNA will be translated into protein.[58] This cascade further intensifies placental dysfunction, insulin resistance, and the progression of GDM. It is worth mentioning that NOS3 is one of the downstream signaling effector in VEGF signaling pathway.[59] A wide group of drugs named anti-VEGF targeting the VEGF signaling pathway and its effector molecules have been used in ophthalmology department.[60] Intravitreal anti-VEGF injections for orbital diseases have been used with caution during pregnancy with acceptable outcome.[61] However, given the complex nature of GDM and maternal-fetal interplay then, systematic administration of anti-VEGF in GDM remained to be investigated.
SHANK2, a scaffolding protein, regulates protein movement in neurons and renal podocytes.[62] SHANK2 knockout mice develop proteinuria, indicating its role in protein trafficking within renal tubules.[63] Bomback and colleagues reported that the incidence of microalbuminuria is higher in pregnant women with GDM compared to those without GDM.[64] In addition, Dehmer et al., found an increased incidence of overt albuminuria in Black pregnant women with GDM, which poses a risk for the development of chronic kidney disease a known diabetes, and GDM complications.[65] Although little is known about the specific role of SHANK2 in GDM, in this study it was found to be hypomethylated and overexpressed, suggesting a potential protective role against the GDM-related complication namely chronic kidney disease.
DPYSL3, another hub gene, encodes dihydropyrimidinase-like 3 protein, a cell adhesion protein linked to metastasis in certain cancers, and based to its action researchers classified it as a tumor suppressor gene.[66] No prior studies have investigated the association between DPYSL3 and GDM, but Umetsu et al. reported that its promoter is hypermethylated in patients with hepatocellular carcinoma and concurrent Type 2 diabetes mellitus.[67] They found also, DPYSL3 downregulation is accompanied with overexpression of VEGF. In this study, DPYSL3 was downregulated and associated with GDM. VEGF, an angiogenesis promoting factor, is linked to hypoxia and placental dysfunction.[68-70] Moreover, VEGF also has a role as inflammatory mediator, contributing to lipid accumulation, adipocyte hypertrophy, and insulin resistance[71] From another perspective, ESRRG was also found in this study as a hub-gene related to GDM and it was hypomethylated and overexpressed. It has been reported that, overexpression of ESRRG in placenta is associated with placenta dysfunction in preeclampsia.[72] It is found that ESRRG expression is affected by the HIF-1α which is the major placenta sensor for hypoxia.[73] Interestingly, ESRRG is also thought to regulate VEGF, however the exact role is controversial.[74] Perhaps, both ESRRG and VEGF synergistically contribute to insulin resistance and placental dysfunction, thereby exacerbating GDM. However, to confirm the association between DPYSL3, VEGF and ESRRG in relation to GDM further studies are needed.
GDPD5 is a hub gene identified in this study and its major function is in the regulation of insulin secretion, glucose metabolism, and insulin signaling.[75] Its overexpression is associated with inhibiting the acetyl-CoA carboxylase enzyme, the main regulatory enzyme in fatty acid synthesis pathway.[76] Furthermore, GDPD5 overexpression is associated with increased accumulation of glycerol-3 phosphate and fatty acids accumulations. Accumulated fatty acids are well associated with the development of insulin resistance a cardinal sign in GDM pathogenicity.[77,78] Yet, The exact role of GDPD5 in GDM needs to be established.
In this study, six genes were identified as hypomethylated-high expression (UCKL1, SHANK2, GDPD5, CMYA5, ESRRG, NOS3) genes, and two hypermethylated-low expression genes (DPYSL3, FTH1). However, the relationship between methylation status and levels of gene expression is not always direct and should be handled with caution.[79] Researchers reported that hypomethylation does not always lead to upregulation of gene expression. There is a consensus that hypomethylation in promoter regions is generally associated with increased gene expression,[80] whereas hypomethylation within transcription sites (gene body) does not necessarily influence expression levels. Conversely, hypermethylation in promoter regions typically suppresses gene expression,[81] while hypermethylation in transcribed regions has been observed to enhance gene expression.[81] This discrepancy can be partially attributed to the affinity of the transcription factors to bind to the promoter region compared to that in the gene body sequences. In the context of GDM,
Over the last two decades, so many epigenetics-targeted drugs have been approved by the Food and Drug Administration to be used in clinical practice, primarily for treating malignant diseases in addition to other conditions.[82] These drugs target various epigenetics enzymes, such as DNA methyltransferase, HDAC, and others.[82] Clinically, these agents have successfully reversed undesired histone modifications and DNA methylation on the targeted genes.[83] Thus epigenetics-targeted drugs have the potential to interrupt the vicious cycle of transgenerational inheritance from mother to the fetus, particularly for metabolic diseases like GDM. However, identifying pharmacological molecules capable of achieving the desired epigenetics-modifications is of paramount importance in the process of developing epigenetics-targeted drug specifically for GDM. This process would involve preclinical studies, including extensive animal testing, to identify its possible teratogenicity and serious side effects, followed by clinical trials to assess therapeutic outcomes in women. Therefore, multi-omics studies are considered as a crucial starting point by providing preliminary data to identify hub genes that could be targeted by epigenetics-targeted drugs.
To conclude, this study employed a multi-omics approach and for the 1st time identified novel GDM-related hub DMGs (UCKL1, SHANK2, GDPD5, CMYA5, ESRRG, NOS3, DPYSL, and FTH1). Three genes (NOS3, ESRRG, and DPYSL) were linked to placental dysfunction, two (UCKL1 and FTH1) were related to ferroptosis pathway, while CMYA5 and SHANK2 were associated with fetal cardiovascular complications and proteinuria. GDPD5 promotes insulin resistance. Investigating epigenetics changes in GDM and identifying GDM-related hub DMGs may provide a pathway to interrupt the transgenerational transmission of GDM and aid in the development of therapeutic or diagnostic approaches targeting these modified genes. The findings of this study should be validated further through RT-qPCR analysis, and further studies are needed.
Author’s Declaration Statements
Ethics approval
This study used secondary data; hence, ethical approval is not needed.
Funding statement
This research received no external funding.
Competing interests
Nothing to declare.
Availability of data and material
The datasets used and/or analyzed during the current study are available within the paper.
Consent for publication
Patient consent was not applicable as open-access secondary data were used.
Authors contributions
HZH solely contributed to the conceptualization of this study, the design of the methodology, formal analysis, and the drafting and review of the manuscript.
References
- Projections of the prevalence of hyperglycaemia in pregnancy in 2019 and beyond:Results from the International Diabetes Federation Diabetes Atlas, 9th edition. Diabetes Res Clin Pract. 2019;157:107841.
- [Google Scholar]
- DNA methylation in gestational diabetes and its predictive value for postpartum glucose disturbances. J Clin Endocrinol Metab. 2022;107:2748-57.
- [Google Scholar]
- Gestational diabetes mellitus epigenetically affects genes predominantly involved in metabolic diseases. Epigenetics. 2013;8:935-43.
- [Google Scholar]
- Effect of treatment of gestational diabetes mellitus on pregnancy outcomes. N Engl J Med. 2005;352:2477-86.
- [Google Scholar]
- A multicenter, randomized trial of treatment for mild gestational diabetes. N Engl J Med. 2009;361:1339-48.
- [Google Scholar]
- Genetics and epigenetics:New insight on gestational diabetes mellitus. Front Endocrinol (Lausanne). 2020;11:602477.
- [Google Scholar]
- Exposure to Gestational Diabetes Mellitus (GDM) alters DNA methylation in placenta and fetal cord blood. Diabetes Res Clin Pract. 2021;174:108690.
- [Google Scholar]
- Maternal gestational diabetes mellitus and newborn DNA methylation:Findings From the pregnancy and childhood epigenetics consortium. Diabetes Care. 2020;43:98-105.
- [Google Scholar]
- The significance of the placental genome and methylome in fetal and maternal health. Hum Genet. 2019;139:1183-96.
- [Google Scholar]
- Epigenetic signatures of gestational diabetes mellitus on cord blood methylation. Clin Epigenetics. 2017;9:28.
- [Google Scholar]
- Chapter 5:Network biology approach to complex diseases. PLoS Comput Biol. 2012;8:e1002820.
- [Google Scholar]
- Identification of hub-methylated differentially expressed genes in patients with gestational diabetes mellitus by multi-omic WGCNA basing epigenome-wide and transcriptome-wide profiling. J Cell Biochem. 2020;121:3173-84.
- [Google Scholar]
- Exposure to gestational diabetes enriches immune-related pathways in the transcriptome and methylome of human amniocytes. J Clin Endocrinol Metab. 2020;105:3250-64.
- [Google Scholar]
- Association of the LEP gene with immune infiltration as a diagnostic biomarker in preeclampsia. Front Mol Biosci. 2023;10:1209144.
- [Google Scholar]
- Human fetoplacental arterial and venous endothelial cells are differentially programmed by gestational diabetes mellitus, resulting in cell-specific barrier function changes. Diabetologia. 2018;61:2398-411.
- [Google Scholar]
- Altered expression of PGC-1a and PDX1 and their methylation status are associated with fetal glucose metabolism in gestational diabetes mellitus. Biochem Biophys Res Commun. 2018;501:300-6.
- [Google Scholar]
- Increased global placental DNA methylation levels are associated with gestational diabetes. Clin Epigenetics. 2016;8:82.
- [Google Scholar]
- Molecular mechanisms of transgenerational epigenetic inheritance. Nat Rev Genet. 2022;23:325-41.
- [Google Scholar]
- Review:Chromatin structural features and targets that regulate transcription. J Struct Biol. 2000;129:102-22.
- [Google Scholar]
- MECHANISMS IN ENDOCRINOLOGY:Epigenetic modifications and gestational diabetes:A systematic review of published literature. Eur J Endocrinol. 2017;176:R247-67.
- [Google Scholar]
- H3K9 and H3K14 acetylation co-occur at many gene regulatory elements, while H3K14ac marks a subset of inactive inducible promoters in mouse embryonic stem cells. BMC Genomics. 2012;13:424.
- [Google Scholar]
- Histone H3 lysine 9 acetylation is downregulated in GDM placentas and calcitriol supplementation enhanced this effect. Int J Mol Sci. 2018;19:4061.
- [Google Scholar]
- Minerals in pregnancy and newborns. In: Molecular Nutrition:Mother and Infant. Amsterdam, Netherlands: Elsevier; 2021. p. :155-77.
- [Google Scholar]
- Association of selenium levels with gestational diabetes mellitus:An updated systematic review and meta-analysis. Nutrients. 2022;14:3941.
- [Google Scholar]
- Relationship of circulating copper level with gestational diabetes mellitus:A meta-analysis and systemic review. Biol Trace Elem Res. 2021;199:4396-409.
- [Google Scholar]
- Selenium supplementation and gestational diabetes:A randomised controlled trial. J Coll Physicians Surg Pak. 2024;34:561-7.
- [Google Scholar]
- The effects of magnesium-zinc-calcium-vitamin D co-supplementation on biomarkers of inflammation, oxidative stress and pregnancy outcomes in gestational diabetes. BMC Pregnancy Childbirth. 2019;19:107.
- [Google Scholar]
- LAIR2 localizes specifically to sites of extravillous trophoblast invasion. Placenta. 2010;31:880-5.
- [Google Scholar]
- Collagens are functional, high affinity ligands for the inhibitory immune receptor LAIR-1. J Exp Med. 2006;203:1419-25.
- [Google Scholar]
- The soluble leukocyte-associated Ig-like receptor (LAIR)-2 antagonizes the collagen/LAIR-1 inhibitory immune interaction. J Immunol. 2008;180:1662-9.
- [Google Scholar]
- A proinflammatory CD4+ T cell phenotype in gestational diabetes mellitus. Diabetologia. 2018;61:1633-43.
- [Google Scholar]
- Gestational diabetes mellitus and maternal immune dysregulation:What we know so far. Int J Mol Sci. 2021;22:4261.
- [Google Scholar]
- Berberine regulates GPX4 to inhibit ferroptosis of islet b cells. Planta Med. 2023;89:254-61.
- [Google Scholar]
- NADPH dynamics:Linking insulin resistance and b-Cells ferroptosis in diabetes mellitus. Int J Mol Sci. 2023;25:342.
- [Google Scholar]
- Bioinformatics-based identification of ferroptosis-related genes and their diagnostic value in gestational diabetes mellitus. Endocr Metab Immune Disord Drug Targets. 2024;24:1611-21.
- [Google Scholar]
- Ferroptosis and its potential role in gestational diabetes mellitus:Updated evidence from pathogenesis to therapy. Front Endocrinol (Lausanne). 2023;14:1177547.
- [Google Scholar]
- Non-canonical role of UCKL1 on ferroptosis defence in colorectal cancer. EBioMedicine. 2023;93:104650.
- [Google Scholar]
- FTH1 inhibits ferroptosis through ferritinophagy in the 6-OHDA model of Parkinson's disease. Neurotherapeutics. 2020;17:1796-812.
- [Google Scholar]
- FTH1- and SAT1-induced astrocytic ferroptosis is involved in Alzheimer's disease:Evidence from single-cell transcriptomic analysis. Pharmaceuticals. 2022;15:1177.
- [Google Scholar]
- Resveratrol inhibits ferroptosis in the lung tissues of heat stroke-induced rats via the Nrf2 pathway. BMC Pharmacol Toxicol. 2024;25:88.
- [Google Scholar]
- Fucoxanthin prevents lipopolysaccharide-induced acute lung injury by inhibiting ferroptosis via Nrf2/STAT3 and glutathione pathways. Am J Chin Med. 2024;52:1773-94.
- [Google Scholar]
- Genetic analysis of common variants in the CMYA5 (cardiomyopathy-associated 5) gene with schizophrenia. Prog Neuropsychopharmacol Biol Psychiatry. 2013;46:64-9.
- [Google Scholar]
- Investigation of pathogenic genes in chinese sporadic hypertrophic cardiomyopathy patients by whole exome sequencing. Sci Rep. 2015;5:16609.
- [Google Scholar]
- Investigating the mechanism of hyperglycemia-induced fetal cardiac hypertrophy. PLoS One. 2015;10:e0139141.
- [Google Scholar]
- Prevalence of hypertrophic cardiomyopathy in fetuses of mothers with gestational diabetes before initiating treatment. Rev Bras Ginecol Obstet. 2017;39:9-13.
- [Google Scholar]
- Association of gestational diabetes mellitus with left ventricular structure and function:The CARDIA study. Diabetes Care. 2016;39:400-7.
- [Google Scholar]
- Fetal myocardial hypertrophy in an experimental model of gestational diabetes. Cardiol Young. 2001;11:609-13.
- [Google Scholar]
- Human umbilical vein endothelium-derived exosomes play a role in foetoplacental endothelial dysfunction in gestational diabetes mellitus. Biochim Biophys Acta Mol Basis Dis. 2018;1864:499-508.
- [Google Scholar]
- The role of maternal gestational diabetes in inducing fetal endothelial dysfunction. J Cell Physiol. 2015;230:2695-705.
- [Google Scholar]
- Fetoplacental endothelial exosomes modulate high d-glucose-induced endothelial dysfunction. Placenta. 2018;66:26-35.
- [Google Scholar]
- Association of gestational diabetes with abnormal maternal vascular endothelial function. Br J Obstet Gynaecol. 1997;104:229-34.
- [Google Scholar]
- Blood pressure and insulin resistance in non-diabetic and normotensive pregnant women. World Acad Sci J. 2024;6:60.
- [Google Scholar]
- Regulation of obesity and insulin resistance by nitric oxide. Free Radic Biol Med. 2014;73:383-99.
- [Google Scholar]
- A data-driven review of the genetic factors of pregnancy complications. Int J Mol Sci. 2020;21:3384.
- [Google Scholar]
- Changes in the TNF-alpha/IL-10 ratio in hyperglycemia-associated pregnancies. Diabetes Res Clin Pract. 2015;107:362-9.
- [Google Scholar]
- Nitric oxide synthase modulates angiogenesis in response to tissue ischemia. J Clin Invest. 1998;101:2567-78.
- [Google Scholar]
- Anti-vascular endothelial growth factor agents for ocular angiogenesis and vascular permeability. J Ophthalmol. 2012;2012:898207.
- [Google Scholar]
- Intravitreal anti-VEGF injections in pregnancy:Case series and review of literature. J Ocul Pharmacol Ther. 2015;31:605-10.
- [Google Scholar]
- Gestational diabetes mellitus alone in the absence of subsequent diabetes is associated with microalbuminuria:Results from the Kidney Early Evaluation Program (KEEP) Diabetes Care. 2010;33:2586-91.
- [Google Scholar]
- Association between gestational diabetes and incident maternal CKD:The coronary artery risk development in young adults (CARDIA) study. Am J Kidney Dis. 2018;71:112-22.
- [Google Scholar]
- Inhibition of cell-adhesion protein DPYSL3 promotes metastasis of lung cancer. Respir Res. 2018;19:41.
- [Google Scholar]
- Diabetes, an independent poor prognostic factor of non-B non-C hepatocellular carcinoma, correlates with dihydropyrimidinase-like 3 promoter methylation. Sci Rep. 2020;10:1156.
- [Google Scholar]
- Placental diabesity:Placental VEGF and CD31 expression according to pregestational BMI and gestational weight gain in women with gestational diabetes. Arch Gynecol Obstet. 2023;307:1823-31.
- [Google Scholar]
- The offspring of the diabetic mother--short- and long-term implications. Best Pract Res Clin Obstet Gynaecol. 2015;29:256-69.
- [Google Scholar]
- Vascular endothelial growth factor (VEGF)-key factor in normal and pathological angiogenesis. Rom J Morphol Embryol. 2018;59:455-67.
- [Google Scholar]
- Transgenic overexpression of VEGF-C induces weight gain and insulin resistance in mice. Sci Rep. 2016;6:31566.
- [Google Scholar]
- Estrogen-related receptor g serves a role in blood pressure homeostasis during pregnancy. Mol Endocrinol. 2014;28:965-75.
- [Google Scholar]
- Estrogen-related receptor gamma (ERRgamma) mediates oxygen-dependent induction of aromatase (CYP19) gene expression during human trophoblast differentiation. Mol Endocrinol. 2011;25:1513-26.
- [Google Scholar]
- The potential role of the E SRRG pathway in placental dysfunction. Reproduction. 2021;161:R45-60.
- [Google Scholar]
- Host genetics and gut microbiota jointly regulate blood biochemical indicators in chickens. Appl Microbiol Biotechnol. 2023;107:7601.
- [Google Scholar]
- GDPD5 related to lipid metabolism is a potential prognostic biomarker in neuroblastoma. Int J Mol Sci. 2022;23:13740.
- [Google Scholar]
- Lipolysis pathways modulate lipid mediator release and endocannabinoid system signaling in dairy cows'adipocytes. J Anim Sci Biotechnol. 2024;15:103.
- [Google Scholar]
- Gene body-specific methylation on the active X chromosome. Science. 2007;315:1141-3.
- [Google Scholar]
- Functions of DNA methylation:Islands, start sites, gene bodies and beyond. Nat Rev Genet. 2012;13:484-92.
- [Google Scholar]
- Gene body methylation can alter gene expression and is a therapeutic target in cancer. Cancer Cell. 2014;26:577-90.
- [Google Scholar]
- Epigenetics-targeted drugs:Current paradigms and future challenges. Signal Transduct Target Ther. 2024;9:332.
- [Google Scholar]
- Clonal heterogeneity of acute myeloid leukemia treated with the IDH2 inhibitor enasidenib. Nat Med. 2018;24:1167-77.
- [Google Scholar]